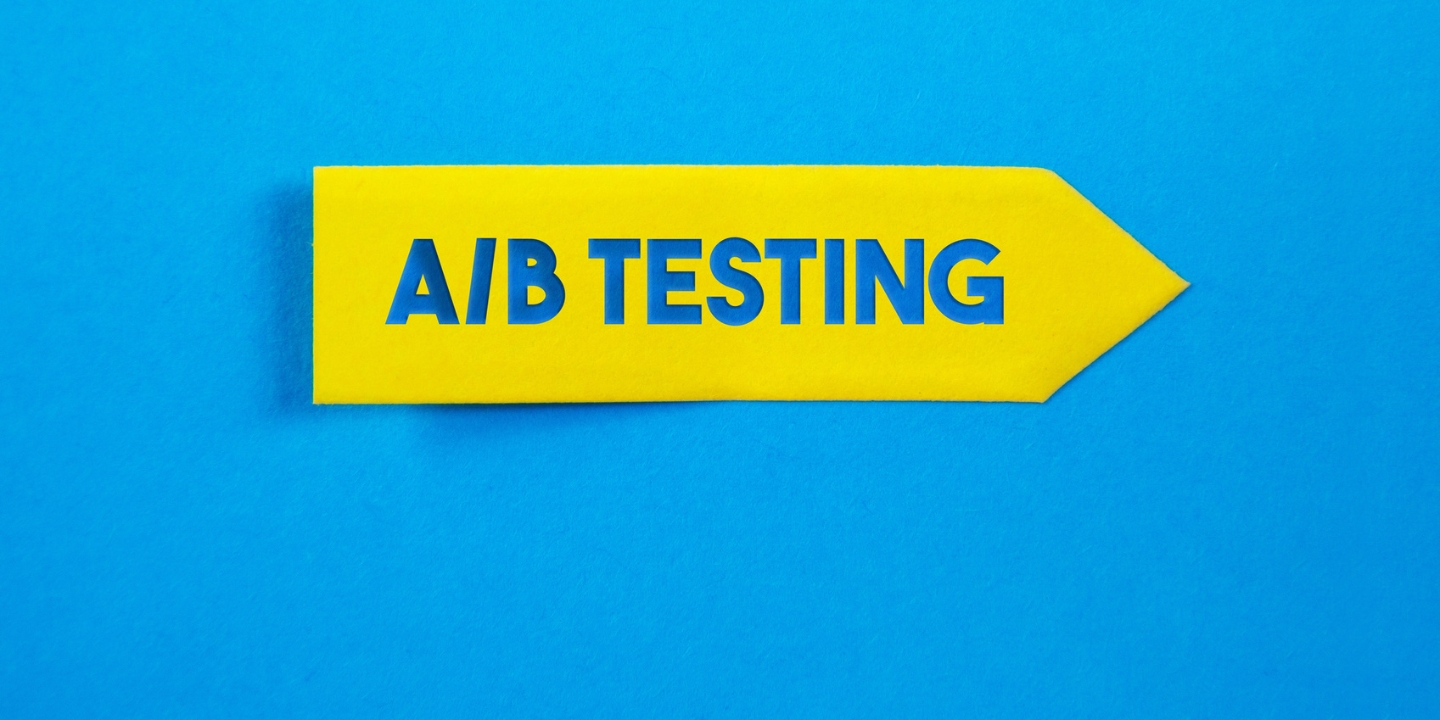
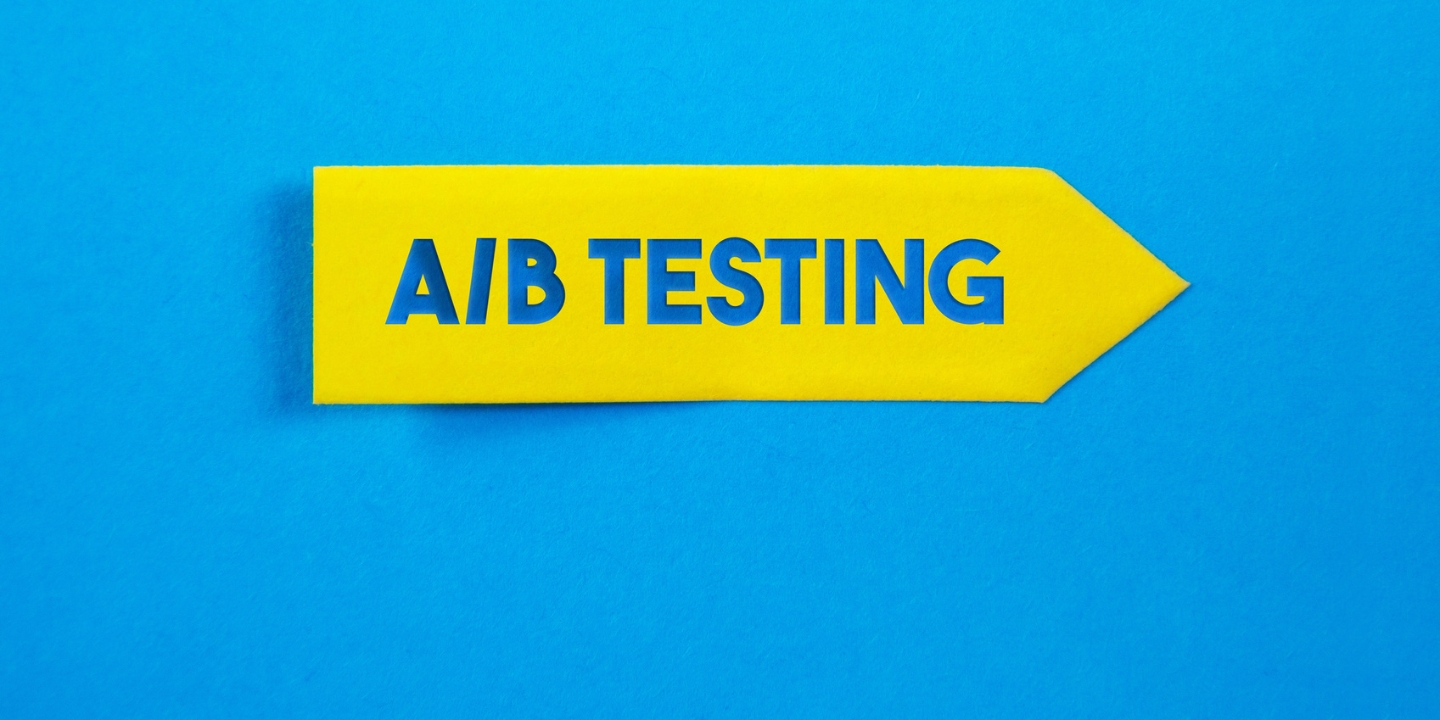
If you are new to AB Testing and just starting your experimentation journey, understanding and interpreting results can be overwhelming. With a number of acronyms and terms in results such as “statistical significance”, “probability density” and “chance to beat control” (The likelihood of observing a particular outcome / real effect and not due to random chance), it can be hard to know what is important.
This article will provide you with helpful information on how to make sense of your AB test results to make sure you are making the right decisions with your data and how to gain further insights
Your sample size explained
Sample size is the number of users that have been bucketed into your control group and your variation group. Without going too technical (we will leave that for the “Common AB testing mistakes” article) sample size is important as it influences the reliability and accuracy of your results.
If you have a larger sample size, you are more likely to observe a statistically significant result, especially on smaller effects.
Remember to calculate your traffic required to observe a Minimum Detectible Effect before the test is started (MDE refers to what is the smallest detectible change compared to the control based on the sample size).
Hello, you need to be a CharityWise member to access the rest of this content.
To join us today, head to our membership page. And if you are not sure if your charity is already a member, then contact us and we can check for you.
If you are a member already, then login below.
Digital toolkit
WordPress glossary (download)
- 8 July 2024
- 5 min read
Local SEO strategies for nonprofit events and
- 22 April 2024
- 4 min read
Campaign tagging for Google Analytics (GA4) –
- 22 April 2024
- 2 min read